A machine learning predictor enhances capability for solving intricate physical problems
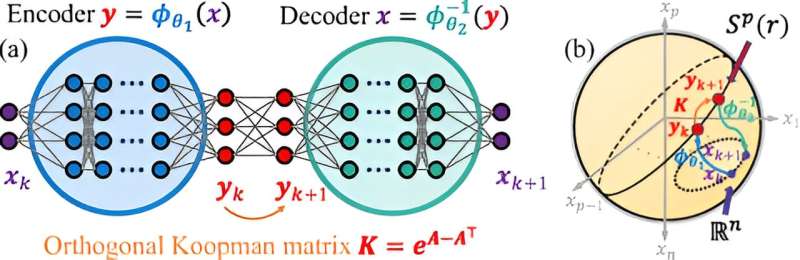
In a recent development at Fudan University, a team of applied mathematicians and AI scientists has unveiled a cutting-edge machine learning framework designed to revolutionize the understanding and prediction of Hamiltonian systems. The paper is in the journal Âé¶¹ÒùÔºical Review Research.
Named the Hamiltonian Neural Koopman Operator (HNKO), this innovative framework integrates principles of mathematical physics to reconstruct and predict Hamiltonian systems of extremely-high dimension using noisy or partially-observed data.
The HNKO framework, equipped with a unitary Koopman structure, has the remarkable ability to discover new conservation laws solely from observational data. This capability addresses a significant challenge in accurately predicting dynamics in the presence of noise perturbations, marking a major breakthrough in the field of Hamiltonian mechanics.
Researchers at Fudan University showcased the power of HNKO and its extensions by applying it to a range of physical models, including celestial n-body systems with hundreds and thousands of degrees of freedom.
Their numerical experiments demonstrated the framework's effectiveness in scaling to complex physical systems, reaffirming its potential to revolutionize the understanding of complex dynamical systems.
This achievement highlights the importance of incorporating prior knowledge and mathematical theory into machine learning frameworks, significantly enhancing their capability to solve intricate physical problems. Fudan University's pioneering work signifies a critical step forward in leveraging artificial intelligence for advancing our understanding of fundamental physics and mathematics.
More information: Jingdong Zhang et al, Learning Hamiltonian neural Koopman operator and simultaneously sustaining and discovering conservation laws, Âé¶¹ÒùÔºical Review Research (2024).
Journal information: Âé¶¹ÒùÔºical Review Research
Provided by Fudan University